Mastering Image Labeling for Object Detection: A Comprehensive Guide
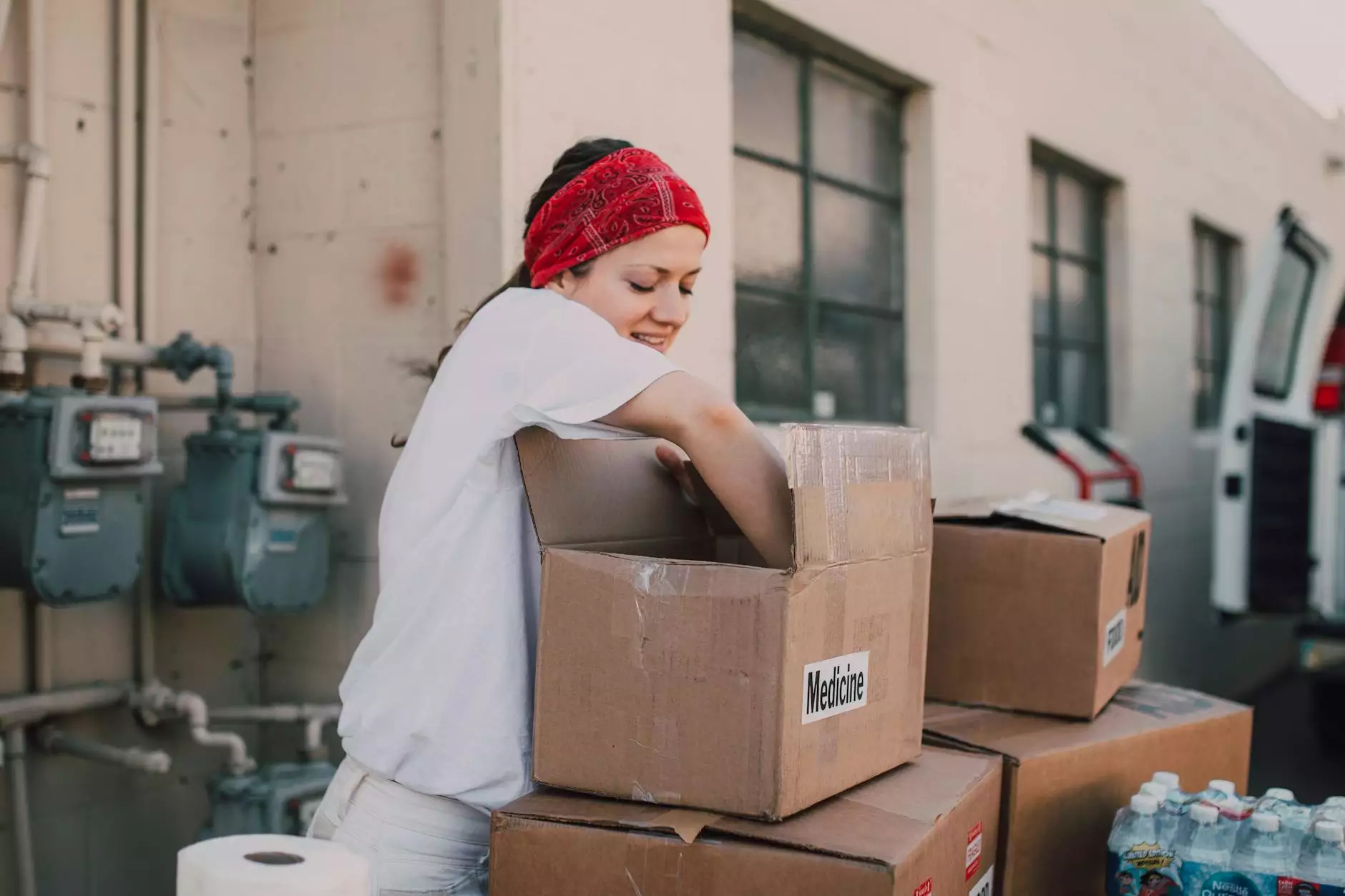
In the rapidly evolving field of artificial intelligence, the ability to label images for object detection has emerged as a crucial component for training machine learning models. As companies like Keylabs.ai strive to provide top-notch data annotation tools and platforms, understanding the intricacies of effective image labeling becomes paramount for developers, data scientists, and businesses alike.
Understanding Object Detection
Object detection is the task of identifying and localizing objects within an image. It goes beyond simple recognition by not only determining what objects are present but also where they are located. This is generally represented through bounding boxes around objects. The prevalence of this technology spans various applications, including:
- Autonomous vehicles
- Facial recognition systems
- Retail analytics
- Medical imaging analysis
- Surveillance and security systems
Importance of Labeling in Object Detection
Labeling images accurately is crucial because it directly affects the performance of object detection models. High-quality annotations ensure that the models learn the desired features and make accurate predictions. There are several types of annotations used in object detection, including:
- Bounding boxes: Enclosures around objects.
- Polygonal segmentation: Precise outlines of objects.
- Keypoint annotations: Marking specific points of interest on objects.
Given the complexity of these tasks, utilizing a reliable data annotation platform like Keylabs.ai can streamline the process and enhance the quality of your labeled datasets.
Challenges in Labeling Images for Object Detection
While the advantages of image labeling are clear, the process is not without its challenges. Some of the common hurdles include:
- Variety of Objects: Different shapes, sizes, and occlusions can complicate the annotation process.
- Consistency: Maintaining uniformity in labeling across large datasets is critical.
- Scalability: Handling large volumes of images efficiently without compromising on quality.
- Quality Control: Implementing verification processes to ensure labeling accuracy.
Best Practices for Labeling Images for Object Detection
To successfully navigate the challenges of image labeling, adhering to best practices is essential. Here are several key strategies:
1. Choose the Right Annotation Tool
Selecting a robust data annotation tool is the first step. A well-designed platform should offer intuitive interfaces, automation features, and seamless integration capabilities.
2. Define Clear Guidelines
Establish comprehensive labeling guidelines that specify how different objects should be annotated. This helps maintain consistency across annotations. Examples include:
- Size and scale rules for bounding boxes.
- Guidance on handling occluded or partially visible objects.
- Standards for labeling similar objects, like differentiating between species in wildlife images.
3. Utilize Automation Features
Take advantage of machine learning algorithms within the annotation tool to expedite the labeling process. Tools that provide smart labeling or pre-labeling assist with rapid initial annotations, significantly reducing manual efforts.
4. Incorporate Active Learning
Active learning allows you to prioritize the most informative images for labeling. This helps in creating a more efficient annotation process and ensures high-quality datasets for model training.
5. Implement Quality Assurance Processes
A solid quality assurance protocol is necessary to verify annotations. This can include:
- Reviewing a random sample of annotations to check for accuracy.
- Implementing a feedback loop where annotators can learn from mistakes.
- Using multiple annotators for challenging cases to ensure reliability.
The Role of Data Annotation Platforms
A sophisticated data annotation platform offers tools and features that simplify the process of labeling images for object detection. Here’s how they enhance the annotation experience:
1. User-Friendly Interfaces
Modern platforms are designed with intuitive interfaces that reduce the learning curve for annotators. This is crucial for onboarding new team members quickly and maintaining productivity.
2. Collaboration Features
Effective collaboration tools allow teams to work together seamlessly, even remotely. Features like commenting and version control ensure that all team members are on the same page.
3. Scalability & Performance
Data annotation platforms are built to scale, enabling businesses to handle large projects without encountering bottlenecks. The ability to manage multiple annotators and track progress is vital for meeting deadlines.
4. Extensive Export Options
Once images are labeled, the ability to export in various formats is essential for integration with machine learning frameworks. Ensure your platform supports required formats like COCO, Pascal VOC, and YOLO.
Conclusion
As the field of artificial intelligence and machine learning continues to flourish, the ability to label images for object detection will remain a cornerstone of development. By adopting robust strategies, utilizing advanced data annotation tools, and adhering to best practices, organizations can improve their annotation quality and optimize their model training processes.
Investing in high-quality annotations not only enhances model performance but also positions businesses at the forefront of innovation. With dedicated resources like those provided by Keylabs.ai, your journey toward achieving optimal results in image labeling becomes simpler and more efficient.
Elevate your AI projects today with exceptional data annotation platforms, and harness the true potential of your data!